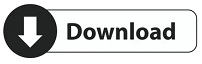
When compared to the control strategy with fixed (nonadapted) parameters, fuel consumption savings between 8 and 11% are reported therein. The authors in exploit the knowledge of an upcoming traffic light location and real-time traffic flow data to anticipate possible upcoming vehicle speed profiles, and thus robustly adapt an energy management strategy of a hybrid electric vehicle. It is shown in that a model predictive controller (MPC) having a control horizon that sees two traffic lights ahead reduces energy consumption by 26% at the expense of a trip time increase of only 1% when compared to a controller with no TLS information.
#DESIGN AN AUTOMATED SHADE CONTROL DRIVER#
The proposed control systems can readily be implemented in future automated driving systems, as well as within advanced driver assist systems such as adaptive cruise control or automated emergency braking systems.Ī vehicle trajectory planning system for approaching a traffic light is often referred to as a green-light optimal speed advisory (GLOSA) system, which utilizes traffic light signal (TLS) information to find an optimal vehicle speed profile to avoid stopping and idling at traffic lights. The performance of the three MPC approaches is compared in terms of vehicle velocity tracking error, root-mean-square acceleration, traveled distance, and computational time. To improve the computational efficiency of the nonlinear MPC formulation, multiple linear MPCs, i.e., a parallel MPC, are designed for different fixed-lag time constants, which can efficiently be solved by fast QP solvers. The nonlinear MPC formulation is based on the first-order lag description of the vehicle velocity profile on the prediction horizon, where only two parameters are optimized: the time constant and the target velocity. To tackle the vehicle-distance-related traffic light constraint, a linear time-varying MPC approach is used. In the linear model predictive control (MPC) case, the vehicle acceleration is optimized at every time instant on a prediction horizon to minimize the root-mean-square error of velocity tracking and RMS acceleration as a comfort metric, thus resulting in a quadratic program (QP). This paper designs and compares different linear and nonlinear model predictive control strategies for a typical scenario of urban driving, in which the vehicle is approaching a traffic light crossing. Taking care of the customer is Lutron’s first principle and our number one priority.Recent advancements in automated driving technology and vehicle connectivity are associated with the development of advanced predictive control systems for improved performance, energy efficiency, safety, and comfort.Whether you are looking to reduce energy costs, increase comfort, or manage light control solutions, Lutron products offer the flexibility you need with the energy savings you want.Ĭonnected lighting, shades, and hand-crafted controls that elevate everyday life.
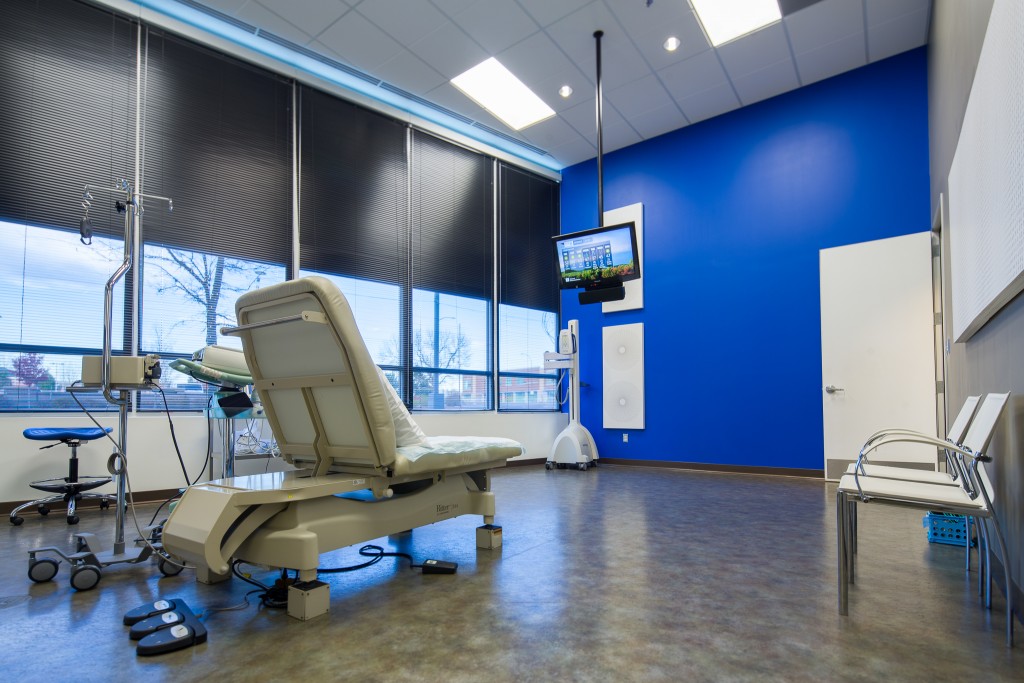

Technical Documentation & Support Overview.
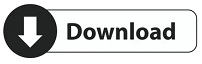